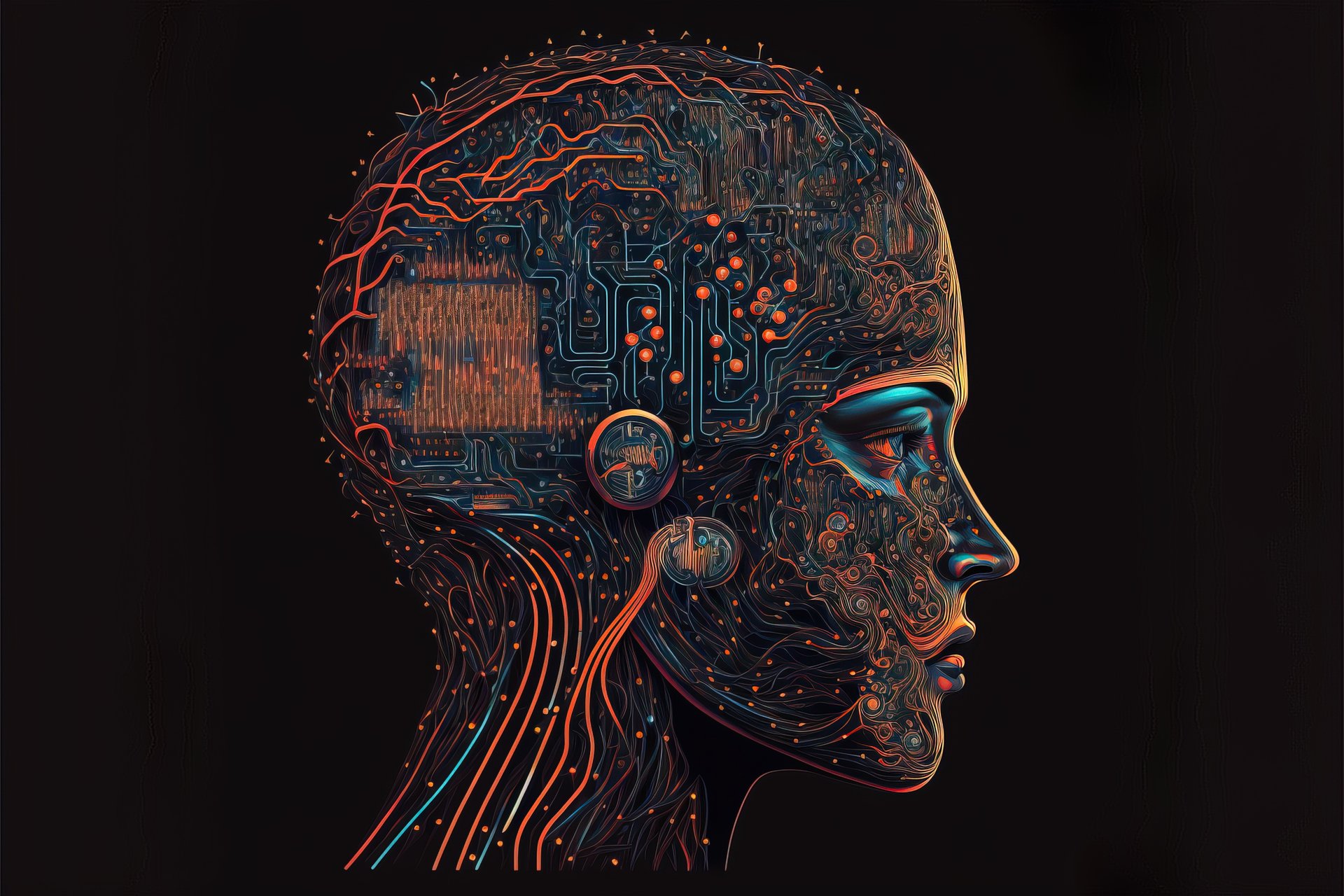
AI Governance
The continued ascendency of artificial intelligence (AI), driven in large part by the growth of generative AI in enterprise solutions, brings about a realization that is more true each day: existing governance and internal control processes must evolve to bring AI-based solutions within their reach before it’s too late. Like incident response, most retroactive risk remediation is incredibly expensive and disruptive to an organization’s operations. As a result, the most prudent approach to safely deploying and maintaining AI-based solutions is to invest in growing your governance program to incorporate these technologies and the unique risks that they bring right now.
A Familiar Approach
It’s not all doom and gloom (read: time and money), as one might think. Despite the novel use cases and technology underlying AI solutions, the approach to governance actually follows similar patterns to more traditional governance risks. All of the following requirements remain the same, even in the AI space:
What data are you collecting and processing?
For what business purposes are you collecting and/or processing that data?
With whom and in what scenarios are you sharing that data (including inferred data and any conclusions generated from initial collection and/or processing)?
For how long will you be retaining that data (including inferred data and any conclusions generated from initial collection and/or processing)?
What data subject rights can/will you support regarding the data being collected/processed?
How will you protect that data while in your control?
For any privacy program, the above questions have become part and parcel to the job regardless of the technology in question. The main difference between traditional technologies and AI-based solution, perhaps, is the complexity involved in answering the above questions definitively.
Layers of Complexity
Where traditional data collection and processing may be focused solely on your organization’s actions, AI-based technologies are increasingly based on foundation data models, like large language models (LLMs), that are developed and curated by third parties. Not only do you have to be concerned with information that your organization collects and processes directly, but also the source of the information against which it is being processed. Put more simply, most AI-based solutions consume or interact with foundational models that are created and maintained by third-parties, which adds more layers of understanding and stewardship than traditional, internally-managed solutions.
The growing depth and breadth of capabilities arising from AI-based solutions makes it far more difficult to nail down the specific business purposes for data consumed by AI-based solutions. Whereas collecting a name and email address in a simple form can be deemed necessary to contact you in response to an inquiry, collection of information for use against AI-based solutions can have a much wider variety of planned and potential use cases in hand.
Identifying the entirety of potential third parties involved in the deployment of AI solutions also creates a layer of complexity. A business not only must consider its direct relationships with third parties in the deployment and management of AI solutions, but also consider the secondary and tertiary relationships that are present in upstream data collection and downstream data usage. This creates a bi- or even omni-directional concern, prompting organizations to more completely understand the entire ecosystem in which they operate and those one or more steps away in relationship networks.
Retention and Protection
The retention of data related to AI-based solutions continues to be heavily debated. For one, the notion of a “right to be forgotten”, as codified by the General Data Protection Regulation (GDPR) and many other global privacy regulations, is something many technologists consider to be impossible when extending obligations to foundational models that have been trained on specific personal information. That is, simply removing a user’s personal information from a dataset doesn’t remove the fact that the model it serves has already trained on and, in a sense, remembered that individual’s information. As a result, understanding what can and cannot be done for your unique AI-based solutions is critical in defining your obligations for continued data subject rights fulfillment. Whether your organization chooses to amend the structure and operations of your solution, or if you simply choose to accept the risk that your model could remember data or signals about individuals after processing it, this is a new and complex consideration that requires depth of thought and insights from leadership and counsel alike.
Protecting the information collected and processed, luckily, mimics the obligations of most traditional data systems and applications. Whether developing preventative, investigative, or corrective controls in your ecosystem, protecting information where it resides and as it moves through your infrastructure remains a very familiar set of requirements.
Steps Forward
With all of the above in mind, what should you be doing today in order to get your arms around the obligations you have (or may have in the near future) as a result of AI-based solutions?
First, you should create and maintain an inventory of systems and applications in use by your organization today that leverage AI-based solutions, techniques, or integrations. Whether through tools that you own or tools that are managed by third parties, each will present unique risks to your organization and to your customers’ data that require your (continued) attention.
Next, categorize those systems and applications according to their purposes for usage, the geographic footprint of data subjects whose data may be involved in each system or application, and the sensitivity of the information and predictive recommendations or decisions being rendered by each system or application. This will help you to prioritize inspection and control deployment in a manner that gives you the most bang for your buck in value-add and risk reduction.
Work directly with the system and application stewards that manage those tools on a day-to-day basis in order to design and implement sustainable control procedures around AI-based solutions. Creating controls in a vacuum, or without taking into account the distinct qualities and use cases of each solution, will likely create undue obstacles for some tools while not fully addressing the risk inherent in others. As such, design and deployment should be informed and based on the unique attributes of each system.
Finally, create monitoring and reporting to keep an eye on how your controls are operating and whether you appear to be mitigating the risk you intended for your organization. Controls for controls’ sake is an unnecessary expense and can slow down your internal processes without garnering a benefit to your risk posture. Controls must be actively monitored to ensure that you are remediating risk as you intend while also protecting the business’s abilities to meet the needs of your customers over time.
It's important to see your AI governance journey as an incremental and iterative one, rather than a point-in-time, one-size-fits-all checklist. Doing so will reduce your monetary impacts, operational disruption, and literal headaches long-term. But, if you’re one of the many seeking to start your journey, or to pivot in a new direction, we’re here to help. Contact our team at Cadence Privacy Consulting to discuss your needs today!